Long before ChatGPT, natural language AI researchers, many of them in academia, shared their research openly. The free-flowing exchange of information and innovation allowed the AI community at large to reproduce, validate, and criticize one another’s work. That all changed with the arrival of supersized LLMs like OpenAI’s GPT-4, when investors started pushing research labs to treat the details of their discoveries as valuable intellectual property—that is, to keep the underlying tech secret.
The Allen Institute for AI (AI2), the Seattle-based nonprofit started by Microsoft’s Paul Allen in 2014, wants to buck that trend. On Thursday, AI2 released a new large language model called OLMo 7B, and shared all the software components and training data that goes with it on GitHub and Hugging Face.
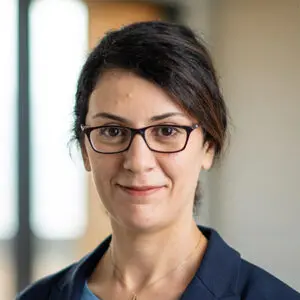
“During this process we actually want to open up everything—the training data, the pretraining data, the source code, the details of the parameters, and so on,” says AI2 senior director of research Hanna Hajishirzi, who leads the OLMo project. “We are also releasing all these intermediate check points that we have obtained throughout training.”
The idea is to give the AI research community full visibility into a state-of-the-art large language model (LLM), which might enable it to advance natural language processing, and confront the problems with existing LLMs in a scientific way.
“We need to put in place a very clear methodology to evaluate how these models are working,” says AI2 COO Sophie Lebrecht, “and the only way to be able to do that is if we have full access to the data, so that we can go back and really understand how the model is behaving.”
In general, AI researchers are still struggling to attribute a specific output by an LLM to a particular piece of training data. Visibility into the reasoning of the model all the way from its training data through its decisions and outputs may help researchers make progress on that front. It could enable progress on other serious problems such as hallucinations and bias.
It’s also true that today’s LLMs are so big—and so expensive to train and operate—that many researchers are forced to use large closed models (via an API) from well-monied players like OpenAI or Google to conduct AI-assisted research. But in doing so they must take the output of those models as-is, with no way of understanding the “why” and “how” of the output.
“Being a researcher in the AI field and just working with APIs or closed models is like being an astronomer trying to research the Solar System and only having access to pictures of it from the newspaper,” Hajishirzi says.
Quoted in the company’s OLMo announcement is Meta chief AI scientist Yann LeCun, an outspoken proponent of open-sourcing new AI models. “The vibrant community that comes from open source is the fastest and most effective way to build the future of AI,” he said in the announcement, echoing a commonly used mantra.
Hajishirzi says Meta’s open-source Llama models have been extremely valuable, but even they aren’t completely open. “They have made the model open but still the data is not available, we don’t understand the connections starting from the data all the way to capabilities,” she says. “Also, the details of the training code is not available. A lot of things are still hidden.”
OLMo is considered a midsized model, with seven billion parameters (the synapse-like connection points in a neural network that contain weighted values). It was trained using two trillion tokens (words, word parts, or phrases).
Lebrecht points out that in an environment where AI researchers keep their discoveries secret, other researchers in academia or in other tech companies often end up trying to reengineer the work. The same work gets repeated, and that has major effects on the amount of power being used to run the servers, and on the carbon effects of that on the environment.
“By opening this up, these different research groups or different companies don’t need to do this siloed research,” Lebrecht says. “So when you open this up, we think it’s going to be huge in decarbonizing the impact of AI.”
Recognize your brand’s excellence by applying to this year’s Brands That Matter Awards before the early-rate deadline, May 3.